Test Your Data Quality to Increase the Return on Your QA Investment
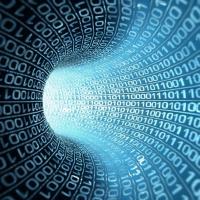
With the high volume of data coming into your organization, it’s important that the information be complete, correct, and timely. The consequences of mismanaged data can be lost revenue opportunities, insufficiently informed decisions, and decreased customer satisfaction.
But considering the velocity at which this data is moving, how do you measure its current quality? You must be able to test it wherever it sits still enough to be viewable, without altering it.
To show data quality (DQ) risks to the decision-makers in your organization, you must be able to retrieve data obtained through these tests and channel it into standard reporting mechanisms. And to quantify the return on investment of your data quality program, you must be able to measure data quality, and any changes in that quality, over time.
To do all of this, you need repeatable, reproducible measures stored in a format easily consumed for reporting and analysis. Data quality testing can provide these measures.
Centralizing the collection and storage of DQ test execution results facilitates automated production monitoring of the health of your data streams, including dashboarding and proactive alert notifications. For instance, automated DQ checks can trigger alerts about issues that require immediate attention, such as runaway conditions, tolerance thresholds being exceeded, or security issues only visible through the data.
Using DQ test execution data to fuel communication and trends analysis in the data flow can lead to measurable process improvements, which can increase the return on investment in your data quality program.
Data quality testing does not reside solely within the realm of software development. You must also test and monitor the quality of your operational data in order to protect your organization from costly errors introduced by factors occurring outside the development cycle. You can do this using a variety of tools that allow reading and sampling of data without altering the data itself.
Regardless of the tool you use, funnel its test execution results into a centralized data quality database. As systems and platforms change, the tools used to view and monitor their data may change. Unifying their output in a single database allows your data quality program to be robust in the face of these changes.
Many tests can be automated and run on a schedule, including source-to-target count checks that monitor ETL processes, domain integrity checks that monitor reference data management, and environment checks that provide snapshots of process lag and operational bottlenecks within the flow of data. These test results can reduce operational costs and avoid progressive degradation of performance:
- Use time-sensitive results data to provide proactive notification alerts when an issue is detected
- Classify results by subject or scope to power dashboards showing the current health of the data system, or identify and quantify the recurrence of chronic issues
- Analyze results over time to identify patterns that point to resource and capacity issues, hardware decay or load balancing problems
To keep your organization running at optimum speed, protect the health of your data by leveraging data quality tests to their fullest.
Shauna Ayers is presenting the session Data Quality at the Speed of Work at the STAREAST 2017 conference, May 7–12 in Orlando, FL.