We Have Tons of Data—Can We Learn Anything from It?
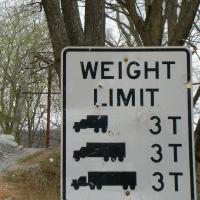
I recently came across a post where the author, Guavus CEO Anukool Lakhina, discussed missing insights in the midst of the “big data avalanche”. It is no secret that we produce more data than ever before.
According to an article in the Harvard Business Review, “As of 2012, about 2.5 exabytes of data are created each day, and that number is doubling every 40 months or so.” Most organizations have understood they need to act on this data, but they struggle with the sheer volume.
One common mistake is to focus on the “Big” part of Big Data, get buried in the avalanche, and fail to find the value. Lakhina provides some very insightful points, and some methods and tools needed to navigate this mass of data. But I want to bring my own perspective.
The answer in creating a proper data analytics structure for your organization could be like any other development endeavor—use agile methodologies. Simply said, it may be best to work in iterations and build out in manageable pieces. Prototyping, QA (data speak “control data”), and allowing constant feedback, which are all part of the agile software development process, also apply here. Many of the lessons we have learned when building software can also be applied to your big data analytics projects.
By working with a small subset initially, you have the advantage of not fighting against these massive volumes while becoming familiar with the data. Analysis can be run quicker, and you can adjust and rerun tests in manageable times. Possible interesting new relationships in the data can be identified and either discarded as not interesting at the moment or alternatively can be incorporated.
Gaps in needed data can be discovered and acted upon more easily, and analytic models can be explored and tested. All of this becomes exponentially harder from an understanding and resource perspective if done with large datasets.
Another way to learn is to make sure you are not just analyzing the data within a silo. While seasoned analysts (or “data scientists” as they are now commonly called) will be the key drivers, other personnel within the organization might find value in the data by looking at it in a new and different way.
The US government is using this method to let people outside of the core government agencies come up with innovations in their data. Of course, you probably don’t want to make your data public like the government is doing, but you can use this model to allow others across your organization play with it and try to discover those hidden gems that can be used in the future.
Once the lessons have been learned, the gates can be opened a little wider and more data allowed in. Repeat until you’re not just storing all of that data but using it to gain insight and value for your organization. By managing and getting to know your data and not rushing to store and analyze everything from the beginning, you will gain long-term benefits.