Business Analysts—Don't Hide from the Data Model
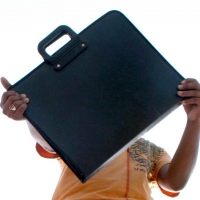
There’s no better way to divide a group of business analysts than to mention data models. As the aptly-named “Business Alchemist” recently observed in his blog, there is often a real reluctance to model data as it is seen as a technical activity rather than a business-focused activity. The Business Alchemist went on to conclude that there is nothing to fear about the “D” word, and this is an area that business analysts should embrace.
I fully support this view. I think it’s so important that project teams gain an understanding of the logical structure of data that an organization is interested in, and the relationships and business rules that should be applied to that data. In fact, there’s no need to refer to it as data modeling. After all, the word data can be scary for our stakeholders, too.
At its heart, data modeling starts by understanding the business concepts we’re interested in. It helps us map out and understand our problem domain and is a great way of generating questions to ask our stakeholders.
Does a customer have one address or many addresses? Can an order have only one delivery address? When you say joint applicant, does this mean there can be one, two, or an infinite number of applicants? All of these are questions that need to be answered before a solution can be designed. Resolving these questions at the requirements stage will help avoid misunderstandings.
Simply sketching out the data model is a great way to generate these questions. It doesn’t need to be technical—and to start with it doesn’t have to be detailed. You don’t need special software; a pen and paper are perfect tools for drafting a model. Loretta Mahon Smith provides a useful overview of some of the key features, benefits, and levels in her “Data Modeling Levels and Styles” presentation.
This type of modeling is a great way to achieve precision and avoid invalid assumptions or misunderstandings. It is exactly these kinds of misunderstandings that lead to increased costs. So spotting a misunderstanding or requirement defect early may reduce the cost to fix it by a factor of ten or even one hundred.
As an example, the challenge that many organizations face in getting a single customer view can, in part, be ascribed to bad data modeling—often combined with a cluttered and diverse IT architecture and projects that were rushed through without a thorough understanding of requirements.
In order to achieve a single customer view, the organization needs to define what a customer is and how this relates to other entities—such as orders, activities, or whatever is relevant for the particular organization.
Data modeling isn’t en vogue and it isn’t trendy—but it is a useful and robust tool when used correctly.